COTADS project blog by Michael Boniface, Director of the IT Innovation Centre | University of Southampton
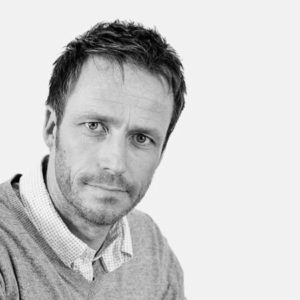
Autonomous systems powered by artificial intelligence (AI) are expected to have a crucial role in the delivery of healthcare and in supporting populations in effective management of disease. Yet, today, digital health solutions insufficiently consider how AI technologies can be successfully integrated into the lives of people living with chronic conditions. As a result, non-adoption and abandonment is common.
The idea that better monitoring and prediction, however accurate, will create improved health outcomes is flawed unless what is learnt by machines can be trusted sufficiently to help empower those with chronic conditions to create positive behavioural change.
Why codesign?
Codesign has been shown to be a powerful way to explore design spaces and foster mutual learning amongst participants.
With everyone treated equally, knowledge and ideas flow between users, data scientists and engineers, improving understanding of data, machine learning, explanations and how models can be aligned with lived experiences and information needs.
This type of understanding has the potential for improving the trustworthiness of human-AI interactions, and therefore improved acceptance, adoption and benefit of digital health interventions.
An integrated methodology of codesign and AI
In the COTADS project, we bring together co-design with explainable AI and provenance to increase accountability, transparency, and trustworthiness of AI models.
The three aspects of COTADS contribute elements necessary for the definition of AI narratives that can improve understanding of AI processes.
Codesign “Participate and Create” provides narrative context through the elaboration of unmet care needs, lived experiences and design of care models into which AI-supported digital interventions will be positioned. Through a range of techniques (e.g. brainstorming, interactive notebooks, interviews) problem statements are explored, domain knowledge shared, interventions designed, hypothesis agreed, results evaluated, and learning facilitated across the team.
Explainable AI “Analyse and Explain” provides narrative evidence of association or causality, including limitations, through data summarisation and visualisation.
Provenance “Track and Record” provides narrative events such as decisions and actions taken within activities that can be combined to explain why an AI process produces specific results, and, increase accountability and traceability of design choices.
Putting the methodology into action: A case study from diabetes
AI has the potential to help people with diabetes navigate uncertainties in chronic conditions, whilst offering decision support to clinicians.
Using AI, patients at most risk can be identified along with contributing critical risk factors. Such information can form the basis for automated personalised recommendations, clinical therapies, and treatments, whilst the awareness of risk can support informed consultation between patients and clinicians.
Through an ongoing series of codesign sessions, supported by novel engagement methods such as computational notebooks, COTADS is helping clinicians, carers and people living with diabetes design better solutions together. The expectation is these future design spaces will allow human learning with machine learning to evolve together in ways that increase engagement, acceptance and efficacy of AI-supported digital health solutions.
Future blogs from the COTADS team will provide a deeper dive into the codesign of machine learning explanations, the use of computational notebooks for codesign, and provenance tracking from design decisions to code.